1244 lines
80 KiB
Plaintext
1244 lines
80 KiB
Plaintext
![]() |
{
|
||
|
"cells": [
|
||
|
{
|
||
|
"cell_type": "markdown",
|
||
|
"metadata": {},
|
||
|
"source": [
|
||
|
"# Elasticsearch Data Analytics\n",
|
||
|
"\n",
|
||
|
"This notebook provides sample code to fetch Elasticsearch Data into and analyze it."
|
||
|
]
|
||
|
},
|
||
|
{
|
||
|
"cell_type": "code",
|
||
|
"execution_count": 1,
|
||
|
"metadata": {},
|
||
|
"outputs": [
|
||
|
{
|
||
|
"data": {
|
||
|
"text/html": [
|
||
|
"<img src=\"https://www.antaresnet.com/wp-content/uploads/2018/07/Elasticsearch-Logo-Color-V.png\"/>"
|
||
|
],
|
||
|
"text/plain": [
|
||
|
"<IPython.core.display.Image object>"
|
||
|
]
|
||
|
},
|
||
|
"execution_count": 1,
|
||
|
"metadata": {},
|
||
|
"output_type": "execute_result"
|
||
|
}
|
||
|
],
|
||
|
"source": [
|
||
|
"from IPython.display import Image\n",
|
||
|
"from IPython.core.display import HTML \n",
|
||
|
"Image(url= \"https://www.antaresnet.com/wp-content/uploads/2018/07/Elasticsearch-Logo-Color-V.png\")"
|
||
|
]
|
||
|
},
|
||
|
{
|
||
|
"cell_type": "markdown",
|
||
|
"metadata": {},
|
||
|
"source": [
|
||
|
"## Loading modules and connect to the Elastic Stack"
|
||
|
]
|
||
|
},
|
||
|
{
|
||
|
"cell_type": "code",
|
||
|
"execution_count": 2,
|
||
|
"metadata": {
|
||
|
"pycharm": {
|
||
|
"is_executing": false
|
||
|
}
|
||
|
},
|
||
|
"outputs": [],
|
||
|
"source": [
|
||
|
"import sys\n",
|
||
|
"import json\n",
|
||
|
"import requests\n",
|
||
|
"import numpy as np\n",
|
||
|
"import pandas as pd\n",
|
||
|
"import seaborn as sns\n",
|
||
|
"import pytz\n",
|
||
|
"from datetime import datetime, timedelta\n",
|
||
|
"from dateutil import tz\n",
|
||
|
"\n",
|
||
|
"import matplotlib.pyplot as plt\n",
|
||
|
"sns.set(style=\"darkgrid\")\n",
|
||
|
"plt.rcParams[\"figure.figsize\"] = (18,10)"
|
||
|
]
|
||
|
},
|
||
|
{
|
||
|
"cell_type": "code",
|
||
|
"execution_count": 3,
|
||
|
"metadata": {
|
||
|
"pycharm": {
|
||
|
"is_executing": false
|
||
|
}
|
||
|
},
|
||
|
"outputs": [
|
||
|
{
|
||
|
"name": "stdout",
|
||
|
"output_type": "stream",
|
||
|
"text": [
|
||
|
"Last run: 2019-12-20 08:12:58.766840 UTC, status: 7.0604683677036 %\n"
|
||
|
]
|
||
|
},
|
||
|
{
|
||
|
"data": {
|
||
|
"text/plain": [
|
||
|
"{'name': 'c185f3ed577c',\n",
|
||
|
" 'cluster_name': 'il.es.cluster',\n",
|
||
|
" 'cluster_uuid': 'sBgbgyRXTvKta2cEJCczKQ',\n",
|
||
|
" 'version': {'number': '6.2.2',\n",
|
||
|
" 'build_hash': '10b1edd',\n",
|
||
|
" 'build_date': '2018-02-16T19:01:30.685723Z',\n",
|
||
|
" 'build_snapshot': False,\n",
|
||
|
" 'lucene_version': '7.2.1',\n",
|
||
|
" 'minimum_wire_compatibility_version': '5.6.0',\n",
|
||
|
" 'minimum_index_compatibility_version': '5.0.0'},\n",
|
||
|
" 'tagline': 'You Know, for Search'}"
|
||
|
]
|
||
|
},
|
||
|
"execution_count": 3,
|
||
|
"metadata": {},
|
||
|
"output_type": "execute_result"
|
||
|
}
|
||
|
],
|
||
|
"source": [
|
||
|
"# connect to our cluster\n",
|
||
|
"from elasticsearch import Elasticsearch\n",
|
||
|
"es = Elasticsearch([{'host': 'elasticsearch', 'port': 9200}])\n",
|
||
|
"print('Last run: {} UTC, status: {} %'.format(\n",
|
||
|
" datetime.utcnow(),\n",
|
||
|
" es.cluster.health()['active_shards_percent_as_number']))\n",
|
||
|
"es.info()"
|
||
|
]
|
||
|
},
|
||
|
{
|
||
|
"cell_type": "markdown",
|
||
|
"metadata": {},
|
||
|
"source": [
|
||
|
"Display our indices and document types saved in elasticsearch.\n",
|
||
|
"\n",
|
||
|
"Update the elasticsearch package."
|
||
|
]
|
||
|
},
|
||
|
{
|
||
|
"cell_type": "code",
|
||
|
"execution_count": 5,
|
||
|
"metadata": {},
|
||
|
"outputs": [
|
||
|
{
|
||
|
"name": "stdout",
|
||
|
"output_type": "stream",
|
||
|
"text": [
|
||
|
"Requirement already satisfied: elasticsearch<8.0.0,>=7.0.0 in /opt/conda/lib/python3.7/site-packages (7.1.0)\n",
|
||
|
"Requirement already satisfied: urllib3>=1.21.1 in /opt/conda/lib/python3.7/site-packages (from elasticsearch<8.0.0,>=7.0.0) (1.25.7)\n"
|
||
|
]
|
||
|
}
|
||
|
],
|
||
|
"source": [
|
||
|
"!sudo pip install \"elasticsearch>=7.0.0,<8.0.0\""
|
||
|
]
|
||
|
},
|
||
|
{
|
||
|
"cell_type": "markdown",
|
||
|
"metadata": {},
|
||
|
"source": [
|
||
|
"## Defining useful functions"
|
||
|
]
|
||
|
},
|
||
|
{
|
||
|
"cell_type": "code",
|
||
|
"execution_count": 6,
|
||
|
"metadata": {},
|
||
|
"outputs": [],
|
||
|
"source": [
|
||
|
"# %load scroller.py\n",
|
||
|
"def scroller(index, quantity, timerange=timedelta(days=0), startdt=\"\", enddt=\"\"):\n",
|
||
|
" print(\"Starting to scroll\", end='')\n",
|
||
|
" # Retrieve the datetimes, note that timerange has a higher priority\n",
|
||
|
" if timerange.total_seconds() > 0:\n",
|
||
|
" now = datetime.utcnow().replace(tzinfo=pytz.UTC)\n",
|
||
|
" startdt = (now - timerange).isoformat()\n",
|
||
|
" enddt = now.isoformat()\n",
|
||
|
" \n",
|
||
|
" # search the first page and write the result to data\n",
|
||
|
" response = es.search(\n",
|
||
|
" index=index,\n",
|
||
|
" body={\n",
|
||
|
" \"query\": {\n",
|
||
|
" \"bool\": {\n",
|
||
|
" \"must\": [\n",
|
||
|
" {\"range\" : {\n",
|
||
|
" \"phenomenonTime\" : {\n",
|
||
|
" #\"gte\": \"2018-02-20T09:08:34.230693+00:00\", \n",
|
||
|
" \"gte\": startdt,\n",
|
||
|
" \"lte\": enddt, \n",
|
||
|
" \"time_zone\": \"+01:00\"\n",
|
||
|
" }\n",
|
||
|
" }},\n",
|
||
|
" {\n",
|
||
|
" \"match_phrase\": {\n",
|
||
|
" \"Datastream.name.keyword\": quantity\n",
|
||
|
" }\n",
|
||
|
" }\n",
|
||
|
" ]\n",
|
||
|
" }\n",
|
||
|
" }\n",
|
||
|
" },\n",
|
||
|
" scroll='10m'\n",
|
||
|
" )\n",
|
||
|
" data = [[row[\"_source\"][\"phenomenonTime\"], row[\"_source\"][\"result\"]] for row in response['hits']['hits']]\n",
|
||
|
"\n",
|
||
|
" # Append new pages until there aren't any left\n",
|
||
|
" while len(response['hits']['hits']):\n",
|
||
|
" print(\".\", end='')\n",
|
||
|
" # process results\n",
|
||
|
" # print([item[\"_id\"] for item in response[\"hits\"][\"hits\"]])\n",
|
||
|
" response = es.scroll(scroll_id=response['_scroll_id'], scroll='10m')\n",
|
||
|
" data += [[row[\"_source\"][\"phenomenonTime\"], row[\"_source\"][\"result\"]] for row in response['hits']['hits']]\n",
|
||
|
" \n",
|
||
|
" # Convert data to a DataFrame and return it\n",
|
||
|
" df = pd.DataFrame(data, columns=[\"phenomenonTime\", quantity])\n",
|
||
|
" # df.index = pd.to_datetime(df[\"phenomenonTime\"].map(lambda t: t.split(\".\")[0]), utc=True)\n",
|
||
|
" df.index = pd.to_datetime(df[\"phenomenonTime\"].map(lambda t: roundto(t, 1)), utc=True)\n",
|
||
|
" df = df.drop([\"phenomenonTime\"], axis=1)\n",
|
||
|
" print(\"\\nFetched {} tuples.\".format(df.shape[0]))\n",
|
||
|
" return df\n",
|
||
|
"\n",
|
||
|
"def roundto(string, n):\n",
|
||
|
" base = string.split(\".\")[0]\n",
|
||
|
" if n > 0:\n",
|
||
|
" base += \".\" + string.split(\".\")[1][:n]\n",
|
||
|
" return base\n"
|
||
|
]
|
||
|
},
|
||
|
{
|
||
|
"cell_type": "markdown",
|
||
|
"metadata": {},
|
||
|
"source": [
|
||
|
"# Gather data from Elasticsearch\n",
|
||
|
"\n",
|
||
|
"It is supposed that in the Elasticsearch instance, there is data with the Datastream.name \"at.srfg.iot-iot4cps-wp5.CarFleet1.car_1.Air Temperature\" within the index name \"at.srfg.iot-iot4cps-wp5.infraprov.internal-*\""
|
||
|
]
|
||
|
},
|
||
|
{
|
||
|
"cell_type": "code",
|
||
|
"execution_count": 7,
|
||
|
"metadata": {},
|
||
|
"outputs": [
|
||
|
{
|
||
|
"name": "stdout",
|
||
|
"output_type": "stream",
|
||
|
"text": [
|
||
|
"Starting to scroll\n",
|
||
|
"Fetched 0 tuples.\n"
|
||
|
]
|
||
|
},
|
||
|
{
|
||
|
"data": {
|
||
|
"text/html": [
|
||
|
"<div>\n",
|
||
|
"<style scoped>\n",
|
||
|
" .dataframe tbody tr th:only-of-type {\n",
|
||
|
" vertical-align: middle;\n",
|
||
|
" }\n",
|
||
|
"\n",
|
||
|
" .dataframe tbody tr th {\n",
|
||
|
" vertical-align: top;\n",
|
||
|
" }\n",
|
||
|
"\n",
|
||
|
" .dataframe thead th {\n",
|
||
|
" text-align: right;\n",
|
||
|
" }\n",
|
||
|
"</style>\n",
|
||
|
"<table border=\"1\" class=\"dataframe\">\n",
|
||
|
" <thead>\n",
|
||
|
" <tr style=\"text-align: right;\">\n",
|
||
|
" <th></th>\n",
|
||
|
" <th>at.srfg.iot-iot4cps-wp5.CarFleet1.car_1.Air Temperature</th>\n",
|
||
|
" </tr>\n",
|
||
|
" <tr>\n",
|
||
|
" <th>phenomenonTime</th>\n",
|
||
|
" <th></th>\n",
|
||
|
" </tr>\n",
|
||
|
" </thead>\n",
|
||
|
" <tbody>\n",
|
||
|
" </tbody>\n",
|
||
|
"</table>\n",
|
||
|
"</div>"
|
||
|
],
|
||
|
"text/plain": [
|
||
|
"Empty DataFrame\n",
|
||
|
"Columns: [at.srfg.iot-iot4cps-wp5.CarFleet1.car_1.Air Temperature]\n",
|
||
|
"Index: []"
|
||
|
]
|
||
|
},
|
||
|
"execution_count": 7,
|
||
|
"metadata": {},
|
||
|
"output_type": "execute_result"
|
||
|
}
|
||
|
],
|
||
|
"source": [
|
||
|
"# Get data for an index and a quantity between two static timestamps\n",
|
||
|
"startdt=\"2019-08-07T08:58:34+00:00\"\n",
|
||
|
"enddt=\"2019-08-07T11:58:34+00:00\"\n",
|
||
|
"df = scroller(\"at.srfg.iot-iot4cps-wp5.infraprov.internal-*\",\n",
|
||
|
" \"at.srfg.iot-iot4cps-wp5.CarFleet1.car_1.Air Temperature\",\n",
|
||
|
" startdt=startdt, enddt=enddt)\n",
|
||
|
"df.head()"
|
||
|
]
|
||
|
},
|
||
|
{
|
||
|
"cell_type": "code",
|
||
|
"execution_count": 12,
|
||
|
"metadata": {},
|
||
|
"outputs": [
|
||
|
{
|
||
|
"name": "stdout",
|
||
|
"output_type": "stream",
|
||
|
"text": [
|
||
|
"Starting to scroll\n",
|
||
|
"Fetched 0 tuples.\n"
|
||
|
]
|
||
|
},
|
||
|
{
|
||
|
"data": {
|
||
|
"text/html": [
|
||
|
"<div>\n",
|
||
|
"<style scoped>\n",
|
||
|
" .dataframe tbody tr th:only-of-type {\n",
|
||
|
" vertical-align: middle;\n",
|
||
|
" }\n",
|
||
|
"\n",
|
||
|
" .dataframe tbody tr th {\n",
|
||
|
" vertical-align: top;\n",
|
||
|
" }\n",
|
||
|
"\n",
|
||
|
" .dataframe thead th {\n",
|
||
|
" text-align: right;\n",
|
||
|
" }\n",
|
||
|
"</style>\n",
|
||
|
"<table border=\"1\" class=\"dataframe\">\n",
|
||
|
" <thead>\n",
|
||
|
" <tr style=\"text-align: right;\">\n",
|
||
|
" <th></th>\n",
|
||
|
" <th>at.srfg.iot-iot4cps-wp5.CarFleet1.car_1.Air Temperature</th>\n",
|
||
|
" </tr>\n",
|
||
|
" <tr>\n",
|
||
|
" <th>phenomenonTime</th>\n",
|
||
|
" <th></th>\n",
|
||
|
" </tr>\n",
|
||
|
" </thead>\n",
|
||
|
" <tbody>\n",
|
||
|
" </tbody>\n",
|
||
|
"</table>\n",
|
||
|
"</div>"
|
||
|
],
|
||
|
"text/plain": [
|
||
|
"Empty DataFrame\n",
|
||
|
"Columns: [at.srfg.iot-iot4cps-wp5.CarFleet1.car_1.Air Temperature]\n",
|
||
|
"Index: []"
|
||
|
]
|
||
|
},
|
||
|
"execution_count": 12,
|
||
|
"metadata": {},
|
||
|
"output_type": "execute_result"
|
||
|
}
|
||
|
],
|
||
|
"source": [
|
||
|
"# Get data for an index and a quantity of the latest timerange\n",
|
||
|
"df = scroller(\"at.srfg.iot-iot4cps-wp5.infraprov.internal-*\",\n",
|
||
|
" \"at.srfg.iot-iot4cps-wp5.CarFleet1.car_1.Air Temperature\",\n",
|
||
|
" timerange=timedelta(days=10))\n",
|
||
|
"df.head()"
|
||
|
]
|
||
|
},
|
||
|
{
|
||
|
"cell_type": "code",
|
||
|
"execution_count": 51,
|
||
|
"metadata": {},
|
||
|
"outputs": [
|
||
|
{
|
||
|
"data": {
|
||
|
"image/png": "iVBORw0KGgoAAAANSUhEUgAABBoAAAI5CAYAAAAPNFn9AAAABHNCSVQICAgIfAhkiAAAAAlwSFlzAAALEgAACxIB0t1+/AAAADl0RVh0U29mdHdhcmUAbWF0cGxvdGxpYiB2ZXJzaW9uIDMuMC4zLCBodHRwOi8vbWF0cGxvdGxpYi5vcmcvnQurowAAIABJREFUeJzs3Xd8VFXCxvFnSnojJAQCIQQSSoBAqIIUwYZSBAQBUSyoqyIiirtrW9vaO4KgoggCIr1XcREUUOmdhNAhAZIQSC+TmfcPJC9IDwl3kvl9/wKSOfeZOZN8uM/cc4/J4XA4BAAAAAAAUALMRgcAAAAAAADlB0UDAAAAAAAoMRQNAAAAAACgxFA0AAAAAACAEkPRAAAAAAAASgxFAwAAAAAAKDEUDQAAAAAAoMRQNAAAAAAAgBJD0QAAAAAAAEoMRQMAAAAAACgxFA0AAAAAAKDEUDQAAAAAAIASYzU6wBlpaVmy2x1Gx8BFBAX5KjU10+gYMAjz79qYf/AecG3Mv2tj/l0b8+/agoJ8lZaWpcBAn6t+rNMUDXa7g6LByTE/ro35d23MP3gPuDbm37Ux/66N+XdtxZ1/lk4AAAAAAIASQ9EAAAAAAABKDEUDAAAAAAAoMU5zjwYAAADg7woLbUpLS5bNlm90FJd0/LhZdrvd6BgwCPPvWqxWdwUGVpLFcu01AUUDAAAAnFZaWrI8Pb3l41NFJpPJ6Dgux2o1y2bjRNNVMf+uw+FwKCsrXWlpyQoODr3m8Vg6AQAAAKdls+XLx8efkgEASpHJZJKPj3+JXT1WYkXDvn371LdvX3Xq1El9+/bV/v37S2poAAAAuDBKBgAofSX5u7bEiobXXntN/fv315IlS9S/f3+9+uqrJTU0AAAAAAAoI0qkaEhNTdWOHTvUtWtXSVLXrl21Y8cOnThxoiSGBwAAAAAAZUSJ3AwyKSlJlStXlsVikSRZLBaFhIQoKSlJFStWLIlDAAAAAE4rKSlRf/75u7p3v/uax5o1a7qmTZssDw8PffHFGHl7+1zR495777+6886uaty4ySW/b+rUH3TbbXcoMPDC/0+fPXu68vLy1LfvfZccZ+XKXxQcHKz69Rue8+8Oh0NDhw5SQkK8Fiz4+YqyG2nDhnX65z+fUfXqNSRJbm5uGjNm/AW/12azady4b7Rs2VJZrVY5HHa1atVGTz75tKzWqzu16t27m9zd3eXu7iFJatq0mYYMGaa3335d9epFq1evvsV+TgsXzlPDho0UHn76OSUnH9ebb/5H8fG7FBYWrm+/nVDssa/FyJGfacWK/ykpKVHff/+jatWKuuT3/+c/L2jjxvWaPXtR0eubkpKsN954RSNGfHXZ47344vNKSkqUJCUkxCsyMkomk1kVK1bUJ5+MvPYndJ0cOXJYGzasU7duPYyOcsWcZteJoCBfoyPgMipV8jM6AgzE/Ls25h+8B1ybkfN//LhZVqvz37/8+PGjmjdvlnr16l3sMWw2m6xWq6ZP/1Gvv/6W6tdvcFWPf+WV167o+6ZOnawbbmilSpWCL/j13r37nPP3i73+v/22QtHR0WrUqNHfxv9RVatWVULC7jIxdxaLWTVr1tK4cZMu+73//e8bysvL0/jxk+Tj4yObrUDz58+V3W6T1ep+Rcc7M8+S9O67Hyoy8tyTbZPJJLPZdE2v3aJF81WxYqBq1aopSfLz89E//vGEsrKy9M03X13V2Feb4+zn93cdOnTUvff21+OPPyKL5dI/26dOndL69X8qPLyG1qz5VR073iJJqlKlskaPHnNFx/7ww0+K/tyqVVONGTNO3t7eV/V8rodLvWaSdOxYkubPn62ePa++yLzc2H9nNpvP+Z1f3PP0EikaQkNDdezYMRUWFspisaiwsFDHjx9XaOiVb4uRmpopu91REnFQCipV8lNycobRMWAQ5t+1Mf/gPeDajJ5/u91etL3eqq1J+m1LUqkcp22jULWJufz/Xd944xUdPHhABQX5qlatul588VX5+/vrww/fU1LSEd1/fz+FhYXprbc+OOdxv/76i8aMGS2z2aLCQpueffZfatq0uQYP/odiYhprx45tcnd3l5eXt44cOazXX39FdetG67XX3tKMGVM0bdqP8vX1U+vWbTRz5tQLXikwePA/dO+9A9SmTTudOJGqDz98V4mJh+VwOHTvvQN0551dNX78t0pJSdaLL/5T7u4eeu21t1SzZq1zxvn226+Uk5OjwYOHymRyaMSI4frjj9WSpBtuuFFPPvm01q37U7/+ukJr1/6hOXNmq2/f/rrzzq46dOigfvppsV566XWtXPnLOVsjzp8/R9Om/Sjp9FUDH3zwqfLy8vToowN0553dtHnzBuXl5WnYsBfUuHETpaWd0Ouvv6K0tFRJUvPmLTVkyLDznvfjjz+soUOfV3R0A3300XvatGmDJk6cKpvNpu7dO2n69PlavnyZli5dJB8fHx0+fFgBAQH6z3/eVKVKISostMvh0GW3cTx06KBWrFiumTMXysPD66/vt6hr156SpLi4eH388XvKzc1Rfn6+7rqrp/r06S9Jevvt1+Xt7a1Dhw7p5Mk0jR07UZJUWGg/77gOh0N2u0M2m10FBQX6+utR2rRpvQoKbIqMjNSwYS/K29tbWVmZGjHiU+3Zs1v5+flq0qS5nn76WS1evEC7du3Qxx9/qC+/HKWnnnpGLVrcoJiYJtqwYd1ln+u2bVv0xRfDlZ2dLZNJGjToGbVs2UojR36mTZs2qKCgQBUqVNCLL76qKlVClZSUqEcfHaC77+6jdev+VKdOd6pHjwsXbg0bNi7684We+9kWLlyg1q3bqGXL1po3b47atesoSUXHO/Mz0LZtcw0aNESrV/+mxo2b6LHHnrzomDbbucdctepXTZjwnfLz8+Xu7q5nnhmm6OgGWrv2D40ePUK1a9fRzp3b5ebmrpdffl1jx36tffv2qEqVqnrnnQ/k4eGpr78epSNHDikzM0vHjiUpIqKWXnrpVXl7+yg/P19fffWFtmzZqPz8AtWuXUfPP/+iPD099eab/5G/f4AOHtyvjIx0jRnzvV577UUdPnxYBQX5ql49XC+88Kr8/Pz04YfvKjn5uO6/v5/Cw2vo1Vf/qw4dWunnn1fJw8NDNput6O8Wi0UdOrTSoEHPaPXqX9W0aXM9/PBjmjDhO/366y+y2WyqXLmK/v3vVy54VZPdbi/6nV+pkp9SUzOLVTaUSNEQFBSk6OhozZ8/X927d9f8+fMVHR3NsgkAAACUK88887wqVKggSfr661GaNGm8nnzyaT333L/0xRfDL3pJ+jfffFV0Al1YWKjc3Jyir+3dm6CPPx5R9Klj797d9NZb76tWrSglJOzWhAnj9N13PygwMFDDh398RTk/++wj1aoVqXff/UgpKSl65JH7VLduPT344COaN2920fiXM3v2TO3eHa+xY09/2v/880M0d+4s9ezZW23btj/nEn+73a73339Lzz337/M+Qd2wYZ0mTPhOo0Z9o6CgYGVnZ8tisSgvL0+nTp1SZGSUBg8eqo0b1+v111/WlCmztXTpIlWpUkXDh4+SJKWnp18wY7NmLbRu3VpFRzfQ1q2b5OHhoZSUFB09mqgaNWrKy8tLkrRly2aNGzdJ4eERGjv2aw0f/lFRIXTo0EENHHifLBar7r77Ht15Z9fzjhMfH6ewsHD5+/tfMEdoaKg++2yU3N3dlZ2drX/840G1bNlaERGnryrYtm2rRo78uiiPJL3yyr+Llk48+eTTuuGG1ueMOWnSePn4+GjMmO8lSaNGfa4JE77T448/pREjPlVsbFO98MJ/ZLfb9cYbr2jBgrm6666eWrRoflHpdDXS00/ppZf+qbff/kAxMY1lMjl06tTpk877739IgwcPlSTNmzdbo0d/rjfeeFfS6asPIiJq6pFHHr+q413KwoVzNXjws2rYMEbDh3+slJRkBQdXuuD32u12jRz59VWNf/DgAU2Y8J0++WSkvL29lZCwWy+88JymT58n6fTP5csvv67IyCh98MHbev75Ifr663EKDq6k554brJ9
|
||
|
"text/plain": [
|
||
|
"<Figure size 1296x720 with 1 Axes>"
|
||
|
]
|
||
|
},
|
||
|
"metadata": {},
|
||
|
"output_type": "display_data"
|
||
|
}
|
||
|
],
|
||
|
"source": [
|
||
|
"# Plot the extracted data using pandas and seaborn\n",
|
||
|
"df.plot()\n",
|
||
|
"plt.show()"
|
||
|
]
|
||
|
},
|
||
|
{
|
||
|
"cell_type": "code",
|
||
|
"execution_count": 52,
|
||
|
"metadata": {},
|
||
|
"outputs": [],
|
||
|
"source": [
|
||
|
"# Get multiple quantities and (outer) join them to a single DataFrame.\n",
|
||
|
"# There can be a lot of missing values\n",
|
||
|
"used_quantities = [\"at.srfg.iot-iot4cps-wp5.CarFleet1.car_1.Air Temperature\", \n",
|
||
|
" \"at.srfg.iot-iot4cps-wp5.CarFleet2.car_2.Air Temperature\"]"
|
||
|
]
|
||
|
},
|
||
|
{
|
||
|
"cell_type": "code",
|
||
|
"execution_count": 53,
|
||
|
"metadata": {},
|
||
|
"outputs": [
|
||
|
{
|
||
|
"name": "stdout",
|
||
|
"output_type": "stream",
|
||
|
"text": [
|
||
|
"Starting to scroll.....\n",
|
||
|
"Fetched 48 tuples.\n",
|
||
|
"at.srfg.iot-iot4cps-wp5.CarFleet2.car_2.Air Temperature\n",
|
||
|
"Starting to scroll\n",
|
||
|
"Fetched 0 tuples.\n"
|
||
|
]
|
||
|
}
|
||
|
],
|
||
|
"source": [
|
||
|
"df = scroller(\"at.srfg.iot-iot4cps-wp5.infraprov.internal-*\",\n",
|
||
|
" used_quantities[0],\n",
|
||
|
" timerange=timedelta(days=10))\n",
|
||
|
"for q in used_quantities[1:]:\n",
|
||
|
" print(q)\n",
|
||
|
" df = df.join(scroller(\"at.srfg.iot-iot4cps-wp5.infraprov.internal-*\", q,\n",
|
||
|
" timerange=timedelta(days=10)),\n",
|
||
|
" how=\"outer\")"
|
||
|
]
|
||
|
},
|
||
|
{
|
||
|
"cell_type": "code",
|
||
|
"execution_count": 54,
|
||
|
"metadata": {},
|
||
|
"outputs": [
|
||
|
{
|
||
|
"data": {
|
||
|
"text/html": [
|
||
|
"<div>\n",
|
||
|
"<style scoped>\n",
|
||
|
" .dataframe tbody tr th:only-of-type {\n",
|
||
|
" vertical-align: middle;\n",
|
||
|
" }\n",
|
||
|
"\n",
|
||
|
" .dataframe tbody tr th {\n",
|
||
|
" vertical-align: top;\n",
|
||
|
" }\n",
|
||
|
"\n",
|
||
|
" .dataframe thead th {\n",
|
||
|
" text-align: right;\n",
|
||
|
" }\n",
|
||
|
"</style>\n",
|
||
|
"<table border=\"1\" class=\"dataframe\">\n",
|
||
|
" <thead>\n",
|
||
|
" <tr style=\"text-align: right;\">\n",
|
||
|
" <th></th>\n",
|
||
|
" <th>at.srfg.iot-iot4cps-wp5.CarFleet1.car_1.Air Temperature</th>\n",
|
||
|
" <th>at.srfg.iot-iot4cps-wp5.CarFleet2.car_2.Air Temperature</th>\n",
|
||
|
" </tr>\n",
|
||
|
" <tr>\n",
|
||
|
" <th>phenomenonTime</th>\n",
|
||
|
" <th></th>\n",
|
||
|
" <th></th>\n",
|
||
|
" </tr>\n",
|
||
|
" </thead>\n",
|
||
|
" <tbody>\n",
|
||
|
" <tr>\n",
|
||
|
" <th>2019-08-07 09:32:50.600000+00:00</th>\n",
|
||
|
" <td>-1.396915</td>\n",
|
||
|
" <td>NaN</td>\n",
|
||
|
" </tr>\n",
|
||
|
" <tr>\n",
|
||
|
" <th>2019-08-07 09:33:40.700000+00:00</th>\n",
|
||
|
" <td>-2.559881</td>\n",
|
||
|
" <td>NaN</td>\n",
|
||
|
" </tr>\n",
|
||
|
" <tr>\n",
|
||
|
" <th>2019-08-07 09:34:10.700000+00:00</th>\n",
|
||
|
" <td>-3.360251</td>\n",
|
||
|
" <td>NaN</td>\n",
|
||
|
" </tr>\n",
|
||
|
" <tr>\n",
|
||
|
" <th>2019-08-07 09:32:10.600000+00:00</th>\n",
|
||
|
" <td>-0.112741</td>\n",
|
||
|
" <td>NaN</td>\n",
|
||
|
" </tr>\n",
|
||
|
" <tr>\n",
|
||
|
" <th>2019-08-07 09:32:40.600000+00:00</th>\n",
|
||
|
" <td>-0.956904</td>\n",
|
||
|
" <td>NaN</td>\n",
|
||
|
" </tr>\n",
|
||
|
" </tbody>\n",
|
||
|
"</table>\n",
|
||
|
"</div>"
|
||
|
],
|
||
|
"text/plain": [
|
||
|
" at.srfg.iot-iot4cps-wp5.CarFleet1.car_1.Air Temperature \\\n",
|
||
|
"phenomenonTime \n",
|
||
|
"2019-08-07 09:32:50.600000+00:00 -1.396915 \n",
|
||
|
"2019-08-07 09:33:40.700000+00:00 -2.559881 \n",
|
||
|
"2019-08-07 09:34:10.700000+00:00 -3.360251 \n",
|
||
|
"2019-08-07 09:32:10.600000+00:00 -0.112741 \n",
|
||
|
"2019-08-07 09:32:40.600000+00:00 -0.956904 \n",
|
||
|
"\n",
|
||
|
" at.srfg.iot-iot4cps-wp5.CarFleet2.car_2.Air Temperature \n",
|
||
|
"phenomenonTime \n",
|
||
|
"2019-08-07 09:32:50.600000+00:00 NaN \n",
|
||
|
"2019-08-07 09:33:40.700000+00:00 NaN \n",
|
||
|
"2019-08-07 09:34:10.700000+00:00 NaN \n",
|
||
|
"2019-08-07 09:32:10.600000+00:00 NaN \n",
|
||
|
"2019-08-07 09:32:40.600000+00:00 NaN "
|
||
|
]
|
||
|
},
|
||
|
"execution_count": 54,
|
||
|
"metadata": {},
|
||
|
"output_type": "execute_result"
|
||
|
}
|
||
|
],
|
||
|
"source": [
|
||
|
"df.head()"
|
||
|
]
|
||
|
},
|
||
|
{
|
||
|
"cell_type": "markdown",
|
||
|
"metadata": {},
|
||
|
"source": [
|
||
|
"## Store and retrieve the DataFrame in a csv"
|
||
|
]
|
||
|
},
|
||
|
{
|
||
|
"cell_type": "code",
|
||
|
"execution_count": 55,
|
||
|
"metadata": {},
|
||
|
"outputs": [],
|
||
|
"source": [
|
||
|
"df.to_csv(\"elasticsearchdata.csv\")"
|
||
|
]
|
||
|
},
|
||
|
{
|
||
|
"cell_type": "code",
|
||
|
"execution_count": 56,
|
||
|
"metadata": {},
|
||
|
"outputs": [
|
||
|
{
|
||
|
"data": {
|
||
|
"text/html": [
|
||
|
"<div>\n",
|
||
|
"<style scoped>\n",
|
||
|
" .dataframe tbody tr th:only-of-type {\n",
|
||
|
" vertical-align: middle;\n",
|
||
|
" }\n",
|
||
|
"\n",
|
||
|
" .dataframe tbody tr th {\n",
|
||
|
" vertical-align: top;\n",
|
||
|
" }\n",
|
||
|
"\n",
|
||
|
" .dataframe thead th {\n",
|
||
|
" text-align: right;\n",
|
||
|
" }\n",
|
||
|
"</style>\n",
|
||
|
"<table border=\"1\" class=\"dataframe\">\n",
|
||
|
" <thead>\n",
|
||
|
" <tr style=\"text-align: right;\">\n",
|
||
|
" <th></th>\n",
|
||
|
" <th>at.srfg.iot-iot4cps-wp5.CarFleet1.car_1.Air Temperature</th>\n",
|
||
|
" <th>at.srfg.iot-iot4cps-wp5.CarFleet2.car_2.Air Temperature</th>\n",
|
||
|
" </tr>\n",
|
||
|
" <tr>\n",
|
||
|
" <th>phenomenonTime</th>\n",
|
||
|
" <th></th>\n",
|
||
|
" <th></th>\n",
|
||
|
" </tr>\n",
|
||
|
" </thead>\n",
|
||
|
" <tbody>\n",
|
||
|
" <tr>\n",
|
||
|
" <th>2019-08-07 09:39:01.100000+00:00</th>\n",
|
||
|
" <td>-3.039692</td>\n",
|
||
|
" <td>NaN</td>\n",
|
||
|
" </tr>\n",
|
||
|
" <tr>\n",
|
||
|
" <th>2019-08-07 09:39:51.100000+00:00</th>\n",
|
||
|
" <td>-1.599475</td>\n",
|
||
|
" <td>NaN</td>\n",
|
||
|
" </tr>\n",
|
||
|
" <tr>\n",
|
||
|
" <th>2019-08-07 09:39:21.100000+00:00</th>\n",
|
||
|
" <td>-2.488179</td>\n",
|
||
|
" <td>NaN</td>\n",
|
||
|
" </tr>\n",
|
||
|
" <tr>\n",
|
||
|
" <th>2019-08-07 09:39:31.100000+00:00</th>\n",
|
||
|
" <td>-2.259640</td>\n",
|
||
|
" <td>NaN</td>\n",
|
||
|
" </tr>\n",
|
||
|
" <tr>\n",
|
||
|
" <th>2019-08-07 09:40:01.200000+00:00</th>\n",
|
||
|
" <td>-1.246612</td>\n",
|
||
|
" <td>NaN</td>\n",
|
||
|
" </tr>\n",
|
||
|
" </tbody>\n",
|
||
|
"</table>\n",
|
||
|
"</div>"
|
||
|
],
|
||
|
"text/plain": [
|
||
|
" at.srfg.iot-iot4cps-wp5.CarFleet1.car_1.Air Temperature \\\n",
|
||
|
"phenomenonTime \n",
|
||
|
"2019-08-07 09:39:01.100000+00:00 -3.039692 \n",
|
||
|
"2019-08-07 09:39:51.100000+00:00 -1.599475 \n",
|
||
|
"2019-08-07 09:39:21.100000+00:00 -2.488179 \n",
|
||
|
"2019-08-07 09:39:31.100000+00:00 -2.259640 \n",
|
||
|
"2019-08-07 09:40:01.200000+00:00 -1.246612 \n",
|
||
|
"\n",
|
||
|
" at.srfg.iot-iot4cps-wp5.CarFleet2.car_2.Air Temperature \n",
|
||
|
"phenomenonTime \n",
|
||
|
"2019-08-07 09:39:01.100000+00:00 NaN \n",
|
||
|
"2019-08-07 09:39:51.100000+00:00 NaN \n",
|
||
|
"2019-08-07 09:39:21.100000+00:00 NaN \n",
|
||
|
"2019-08-07 09:39:31.100000+00:00 NaN \n",
|
||
|
"2019-08-07 09:40:01.200000+00:00 NaN "
|
||
|
]
|
||
|
},
|
||
|
"execution_count": 56,
|
||
|
"metadata": {},
|
||
|
"output_type": "execute_result"
|
||
|
}
|
||
|
],
|
||
|
"source": [
|
||
|
"df = pd.read_csv(\"elasticsearchdata.csv\", parse_dates=True, index_col='phenomenonTime')\n",
|
||
|
"df.tail()"
|
||
|
]
|
||
|
},
|
||
|
{
|
||
|
"cell_type": "markdown",
|
||
|
"metadata": {},
|
||
|
"source": [
|
||
|
"# Pre-processing"
|
||
|
]
|
||
|
},
|
||
|
{
|
||
|
"cell_type": "markdown",
|
||
|
"metadata": {},
|
||
|
"source": [
|
||
|
"## Reduce size and interpolate"
|
||
|
]
|
||
|
},
|
||
|
{
|
||
|
"cell_type": "code",
|
||
|
"execution_count": 57,
|
||
|
"metadata": {},
|
||
|
"outputs": [],
|
||
|
"source": [
|
||
|
"df = pd.read_csv(\"elasticsearchdata.csv\", parse_dates=True, index_col='phenomenonTime')"
|
||
|
]
|
||
|
},
|
||
|
{
|
||
|
"cell_type": "code",
|
||
|
"execution_count": 58,
|
||
|
"metadata": {},
|
||
|
"outputs": [],
|
||
|
"source": [
|
||
|
"df.index.names = [\"time\"]"
|
||
|
]
|
||
|
},
|
||
|
{
|
||
|
"cell_type": "code",
|
||
|
"execution_count": 59,
|
||
|
"metadata": {},
|
||
|
"outputs": [],
|
||
|
"source": [
|
||
|
"col_mapping = {\"at.srfg.iot-iot4cps-wp5.CarFleet1.car_1.Air Temperature\": \"car1_temp\", \n",
|
||
|
" \"at.srfg.iot-iot4cps-wp5.CarFleet2.car_2.Air Temperature\": \"car2_temp\"}\n",
|
||
|
"df = df.rename(index=str, \n",
|
||
|
" columns=col_mapping)"
|
||
|
]
|
||
|
},
|
||
|
{
|
||
|
"cell_type": "code",
|
||
|
"execution_count": 60,
|
||
|
"metadata": {},
|
||
|
"outputs": [
|
||
|
{
|
||
|
"data": {
|
||
|
"text/html": [
|
||
|
"<div>\n",
|
||
|
"<style scoped>\n",
|
||
|
" .dataframe tbody tr th:only-of-type {\n",
|
||
|
" vertical-align: middle;\n",
|
||
|
" }\n",
|
||
|
"\n",
|
||
|
" .dataframe tbody tr th {\n",
|
||
|
" vertical-align: top;\n",
|
||
|
" }\n",
|
||
|
"\n",
|
||
|
" .dataframe thead th {\n",
|
||
|
" text-align: right;\n",
|
||
|
" }\n",
|
||
|
"</style>\n",
|
||
|
"<table border=\"1\" class=\"dataframe\">\n",
|
||
|
" <thead>\n",
|
||
|
" <tr style=\"text-align: right;\">\n",
|
||
|
" <th></th>\n",
|
||
|
" <th>car1_temp</th>\n",
|
||
|
" <th>car2_temp</th>\n",
|
||
|
" </tr>\n",
|
||
|
" <tr>\n",
|
||
|
" <th>time</th>\n",
|
||
|
" <th></th>\n",
|
||
|
" <th></th>\n",
|
||
|
" </tr>\n",
|
||
|
" </thead>\n",
|
||
|
" <tbody>\n",
|
||
|
" <tr>\n",
|
||
|
" <th>2019-08-07 09:32:50.600000+00:00</th>\n",
|
||
|
" <td>-1.396915</td>\n",
|
||
|
" <td>NaN</td>\n",
|
||
|
" </tr>\n",
|
||
|
" <tr>\n",
|
||
|
" <th>2019-08-07 09:33:40.700000+00:00</th>\n",
|
||
|
" <td>-2.559881</td>\n",
|
||
|
" <td>NaN</td>\n",
|
||
|
" </tr>\n",
|
||
|
" <tr>\n",
|
||
|
" <th>2019-08-07 09:34:10.700000+00:00</th>\n",
|
||
|
" <td>-3.360251</td>\n",
|
||
|
" <td>NaN</td>\n",
|
||
|
" </tr>\n",
|
||
|
" <tr>\n",
|
||
|
" <th>2019-08-07 09:32:10.600000+00:00</th>\n",
|
||
|
" <td>-0.112741</td>\n",
|
||
|
" <td>NaN</td>\n",
|
||
|
" </tr>\n",
|
||
|
" <tr>\n",
|
||
|
" <th>2019-08-07 09:32:40.600000+00:00</th>\n",
|
||
|
" <td>-0.956904</td>\n",
|
||
|
" <td>NaN</td>\n",
|
||
|
" </tr>\n",
|
||
|
" </tbody>\n",
|
||
|
"</table>\n",
|
||
|
"</div>"
|
||
|
],
|
||
|
"text/plain": [
|
||
|
" car1_temp car2_temp\n",
|
||
|
"time \n",
|
||
|
"2019-08-07 09:32:50.600000+00:00 -1.396915 NaN\n",
|
||
|
"2019-08-07 09:33:40.700000+00:00 -2.559881 NaN\n",
|
||
|
"2019-08-07 09:34:10.700000+00:00 -3.360251 NaN\n",
|
||
|
"2019-08-07 09:32:10.600000+00:00 -0.112741 NaN\n",
|
||
|
"2019-08-07 09:32:40.600000+00:00 -0.956904 NaN"
|
||
|
]
|
||
|
},
|
||
|
"execution_count": 60,
|
||
|
"metadata": {},
|
||
|
"output_type": "execute_result"
|
||
|
}
|
||
|
],
|
||
|
"source": [
|
||
|
"df.head()"
|
||
|
]
|
||
|
},
|
||
|
{
|
||
|
"cell_type": "code",
|
||
|
"execution_count": 61,
|
||
|
"metadata": {},
|
||
|
"outputs": [],
|
||
|
"source": [
|
||
|
"# Interpolate forwards and backwaonly up to \n",
|
||
|
"df = df.interpolate(method ='linear', limit_direction ='both', limit=10)\n",
|
||
|
"df = df.interpolate(method ='linear', limit_direction ='both', limit=10)"
|
||
|
]
|
||
|
},
|
||
|
{
|
||
|
"cell_type": "code",
|
||
|
"execution_count": 62,
|
||
|
"metadata": {},
|
||
|
"outputs": [],
|
||
|
"source": [
|
||
|
"# Keep only the rows with at least 2 non-NA values.\n",
|
||
|
"df = df.dropna(thresh=2)"
|
||
|
]
|
||
|
},
|
||
|
{
|
||
|
"cell_type": "code",
|
||
|
"execution_count": 63,
|
||
|
"metadata": {},
|
||
|
"outputs": [],
|
||
|
"source": [
|
||
|
"# make Timestamp unique\n",
|
||
|
"df = df.reset_index()\n",
|
||
|
"df = df.groupby(\"time\").agg({q: \"mean\" for q in col_mapping.values()})"
|
||
|
]
|
||
|
},
|
||
|
{
|
||
|
"cell_type": "code",
|
||
|
"execution_count": 64,
|
||
|
"metadata": {},
|
||
|
"outputs": [],
|
||
|
"source": [
|
||
|
"# Interpolate again to close gaps, use the smalles value \n",
|
||
|
"df = df.interpolate(method ='zero', limit_direction ='forward')\n",
|
||
|
"df = df.interpolate(method ='zero', limit_direction ='forward')"
|
||
|
]
|
||
|
},
|
||
|
{
|
||
|
"cell_type": "code",
|
||
|
"execution_count": 65,
|
||
|
"metadata": {},
|
||
|
"outputs": [
|
||
|
{
|
||
|
"data": {
|
||
|
"text/plain": [
|
||
|
"nan"
|
||
|
]
|
||
|
},
|
||
|
"execution_count": 65,
|
||
|
"metadata": {},
|
||
|
"output_type": "execute_result"
|
||
|
}
|
||
|
],
|
||
|
"source": [
|
||
|
"df.index.min()"
|
||
|
]
|
||
|
},
|
||
|
{
|
||
|
"cell_type": "code",
|
||
|
"execution_count": 66,
|
||
|
"metadata": {},
|
||
|
"outputs": [
|
||
|
{
|
||
|
"data": {
|
||
|
"text/plain": [
|
||
|
"(0, 2)"
|
||
|
]
|
||
|
},
|
||
|
"execution_count": 66,
|
||
|
"metadata": {},
|
||
|
"output_type": "execute_result"
|
||
|
}
|
||
|
],
|
||
|
"source": [
|
||
|
"df.shape"
|
||
|
]
|
||
|
},
|
||
|
{
|
||
|
"cell_type": "code",
|
||
|
"execution_count": 67,
|
||
|
"metadata": {},
|
||
|
"outputs": [
|
||
|
{
|
||
|
"data": {
|
||
|
"text/plain": [
|
||
|
"car1_temp 0\n",
|
||
|
"car2_temp 0\n",
|
||
|
"dtype: int64"
|
||
|
]
|
||
|
},
|
||
|
"execution_count": 67,
|
||
|
"metadata": {},
|
||
|
"output_type": "execute_result"
|
||
|
}
|
||
|
],
|
||
|
"source": [
|
||
|
"df.isna().sum()"
|
||
|
]
|
||
|
},
|
||
|
{
|
||
|
"cell_type": "code",
|
||
|
"execution_count": 68,
|
||
|
"metadata": {},
|
||
|
"outputs": [
|
||
|
{
|
||
|
"data": {
|
||
|
"text/html": [
|
||
|
"<div>\n",
|
||
|
"<style scoped>\n",
|
||
|
" .dataframe tbody tr th:only-of-type {\n",
|
||
|
" vertical-align: middle;\n",
|
||
|
" }\n",
|
||
|
"\n",
|
||
|
" .dataframe tbody tr th {\n",
|
||
|
" vertical-align: top;\n",
|
||
|
" }\n",
|
||
|
"\n",
|
||
|
" .dataframe thead th {\n",
|
||
|
" text-align: right;\n",
|
||
|
" }\n",
|
||
|
"</style>\n",
|
||
|
"<table border=\"1\" class=\"dataframe\">\n",
|
||
|
" <thead>\n",
|
||
|
" <tr style=\"text-align: right;\">\n",
|
||
|
" <th></th>\n",
|
||
|
" <th>car1_temp</th>\n",
|
||
|
" <th>car2_temp</th>\n",
|
||
|
" </tr>\n",
|
||
|
" </thead>\n",
|
||
|
" <tbody>\n",
|
||
|
" <tr>\n",
|
||
|
" <th>count</th>\n",
|
||
|
" <td>0.0</td>\n",
|
||
|
" <td>0.0</td>\n",
|
||
|
" </tr>\n",
|
||
|
" <tr>\n",
|
||
|
" <th>mean</th>\n",
|
||
|
" <td>NaN</td>\n",
|
||
|
" <td>NaN</td>\n",
|
||
|
" </tr>\n",
|
||
|
" <tr>\n",
|
||
|
" <th>std</th>\n",
|
||
|
" <td>NaN</td>\n",
|
||
|
" <td>NaN</td>\n",
|
||
|
" </tr>\n",
|
||
|
" <tr>\n",
|
||
|
" <th>min</th>\n",
|
||
|
" <td>NaN</td>\n",
|
||
|
" <td>NaN</td>\n",
|
||
|
" </tr>\n",
|
||
|
" <tr>\n",
|
||
|
" <th>25%</th>\n",
|
||
|
" <td>NaN</td>\n",
|
||
|
" <td>NaN</td>\n",
|
||
|
" </tr>\n",
|
||
|
" <tr>\n",
|
||
|
" <th>50%</th>\n",
|
||
|
" <td>NaN</td>\n",
|
||
|
" <td>NaN</td>\n",
|
||
|
" </tr>\n",
|
||
|
" <tr>\n",
|
||
|
" <th>75%</th>\n",
|
||
|
" <td>NaN</td>\n",
|
||
|
" <td>NaN</td>\n",
|
||
|
" </tr>\n",
|
||
|
" <tr>\n",
|
||
|
" <th>max</th>\n",
|
||
|
" <td>NaN</td>\n",
|
||
|
" <td>NaN</td>\n",
|
||
|
" </tr>\n",
|
||
|
" </tbody>\n",
|
||
|
"</table>\n",
|
||
|
"</div>"
|
||
|
],
|
||
|
"text/plain": [
|
||
|
" car1_temp car2_temp\n",
|
||
|
"count 0.0 0.0\n",
|
||
|
"mean NaN NaN\n",
|
||
|
"std NaN NaN\n",
|
||
|
"min NaN NaN\n",
|
||
|
"25% NaN NaN\n",
|
||
|
"50% NaN NaN\n",
|
||
|
"75% NaN NaN\n",
|
||
|
"max NaN NaN"
|
||
|
]
|
||
|
},
|
||
|
"execution_count": 68,
|
||
|
"metadata": {},
|
||
|
"output_type": "execute_result"
|
||
|
}
|
||
|
],
|
||
|
"source": [
|
||
|
"df.describe()"
|
||
|
]
|
||
|
},
|
||
|
{
|
||
|
"cell_type": "code",
|
||
|
"execution_count": 69,
|
||
|
"metadata": {},
|
||
|
"outputs": [],
|
||
|
"source": [
|
||
|
"# Keep only rows with all filled rows\n",
|
||
|
"df = df.dropna()"
|
||
|
]
|
||
|
},
|
||
|
{
|
||
|
"cell_type": "code",
|
||
|
"execution_count": 70,
|
||
|
"metadata": {},
|
||
|
"outputs": [],
|
||
|
"source": [
|
||
|
"df.to_csv(\"elasticsearchdata.csv\")"
|
||
|
]
|
||
|
},
|
||
|
{
|
||
|
"cell_type": "markdown",
|
||
|
"metadata": {},
|
||
|
"source": [
|
||
|
"# Basic Data Analysis"
|
||
|
]
|
||
|
},
|
||
|
{
|
||
|
"cell_type": "code",
|
||
|
"execution_count": 71,
|
||
|
"metadata": {},
|
||
|
"outputs": [
|
||
|
{
|
||
|
"data": {
|
||
|
"text/html": [
|
||
|
"<div>\n",
|
||
|
"<style scoped>\n",
|
||
|
" .dataframe tbody tr th:only-of-type {\n",
|
||
|
" vertical-align: middle;\n",
|
||
|
" }\n",
|
||
|
"\n",
|
||
|
" .dataframe tbody tr th {\n",
|
||
|
" vertical-align: top;\n",
|
||
|
" }\n",
|
||
|
"\n",
|
||
|
" .dataframe thead th {\n",
|
||
|
" text-align: right;\n",
|
||
|
" }\n",
|
||
|
"</style>\n",
|
||
|
"<table border=\"1\" class=\"dataframe\">\n",
|
||
|
" <thead>\n",
|
||
|
" <tr style=\"text-align: right;\">\n",
|
||
|
" <th></th>\n",
|
||
|
" <th>car1_temp</th>\n",
|
||
|
" <th>car2_temp</th>\n",
|
||
|
" </tr>\n",
|
||
|
" <tr>\n",
|
||
|
" <th>time</th>\n",
|
||
|
" <th></th>\n",
|
||
|
" <th></th>\n",
|
||
|
" </tr>\n",
|
||
|
" </thead>\n",
|
||
|
" <tbody>\n",
|
||
|
" </tbody>\n",
|
||
|
"</table>\n",
|
||
|
"</div>"
|
||
|
],
|
||
|
"text/plain": [
|
||
|
"Empty DataFrame\n",
|
||
|
"Columns: [car1_temp, car2_temp]\n",
|
||
|
"Index: []"
|
||
|
]
|
||
|
},
|
||
|
"execution_count": 71,
|
||
|
"metadata": {},
|
||
|
"output_type": "execute_result"
|
||
|
}
|
||
|
],
|
||
|
"source": [
|
||
|
"df = pd.read_csv(\"elasticsearchdata.csv\", parse_dates=True, index_col='time')\n",
|
||
|
"df.tail()"
|
||
|
]
|
||
|
},
|
||
|
{
|
||
|
"cell_type": "code",
|
||
|
"execution_count": 72,
|
||
|
"metadata": {},
|
||
|
"outputs": [],
|
||
|
"source": [
|
||
|
"# df.hist()\n",
|
||
|
"plt.show()"
|
||
|
]
|
||
|
},
|
||
|
{
|
||
|
"cell_type": "code",
|
||
|
"execution_count": 73,
|
||
|
"metadata": {},
|
||
|
"outputs": [],
|
||
|
"source": [
|
||
|
"# pd.plotting.scatter_matrix(df, alpha=0.2)\n",
|
||
|
"plt.show()"
|
||
|
]
|
||
|
},
|
||
|
{
|
||
|
"cell_type": "code",
|
||
|
"execution_count": 74,
|
||
|
"metadata": {},
|
||
|
"outputs": [],
|
||
|
"source": [
|
||
|
"# corr = df.corr() \n",
|
||
|
"cm = sns.light_palette(\"orange\", as_cmap=True) \n",
|
||
|
"cm = sns.diverging_palette(220, 20, sep=20, as_cmap=True) \n",
|
||
|
"# corr.style.background_gradient(cmap=cm).set_precision(2)"
|
||
|
]
|
||
|
},
|
||
|
{
|
||
|
"cell_type": "markdown",
|
||
|
"metadata": {},
|
||
|
"source": [
|
||
|
"# Feature Engineering\n",
|
||
|
"\n",
|
||
|
"This task is very domain-specific and must be done by an expert."
|
||
|
]
|
||
|
},
|
||
|
{
|
||
|
"cell_type": "markdown",
|
||
|
"metadata": {},
|
||
|
"source": [
|
||
|
"# Data Analytics"
|
||
|
]
|
||
|
},
|
||
|
{
|
||
|
"cell_type": "code",
|
||
|
"execution_count": 77,
|
||
|
"metadata": {},
|
||
|
"outputs": [
|
||
|
{
|
||
|
"data": {
|
||
|
"application/vnd.jupyter.widget-view+json": {
|
||
|
"model_id": "75cfeeea6204489e945d894895b2bc30",
|
||
|
"version_major": 2,
|
||
|
"version_minor": 0
|
||
|
},
|
||
|
"text/plain": [
|
||
|
"interactive(children=(IntSlider(value=45, description='pitch', max=90), IntSlider(value=45, description='yaw',…"
|
||
|
]
|
||
|
},
|
||
|
"metadata": {},
|
||
|
"output_type": "display_data"
|
||
|
}
|
||
|
],
|
||
|
"source": [
|
||
|
"from IPython.html.widgets import *\n",
|
||
|
"from mpl_toolkits.mplot3d import Axes3D\n",
|
||
|
"\n",
|
||
|
"plt.rcParams[\"figure.figsize\"] = (18,10)\n",
|
||
|
"sns.set(style=\"darkgrid\")\n",
|
||
|
"\n",
|
||
|
"def plot3D(pitch, yaw):\n",
|
||
|
" fig = plt.figure()\n",
|
||
|
" ax = fig.add_subplot(111, projection='3d')\n",
|
||
|
" plot = ax.scatter(df['car1_temp'], df['car1_temp'], df['car2_temp'], c=df[\"car1_temp\"], s=60)\n",
|
||
|
" fig.colorbar(plot)\n",
|
||
|
" ax.view_init(pitch, yaw)\n",
|
||
|
" ax.legend(['Vibration for each 3D position'])\n",
|
||
|
" ax.set_xlabel(\"x-Position\")\n",
|
||
|
" ax.set_ylabel(\"y-Position\")\n",
|
||
|
" ax.set_zlabel(\"z-Position\")\n",
|
||
|
"interact(plot3D, pitch=(0,90,1), yaw=(0,90,1))\n",
|
||
|
"plt.show()"
|
||
|
]
|
||
|
},
|
||
|
{
|
||
|
"cell_type": "code",
|
||
|
"execution_count": 78,
|
||
|
"metadata": {},
|
||
|
"outputs": [],
|
||
|
"source": [
|
||
|
"# df[col_mapping.values()].hist()\n",
|
||
|
"plt.show()"
|
||
|
]
|
||
|
},
|
||
|
{
|
||
|
"cell_type": "code",
|
||
|
"execution_count": 79,
|
||
|
"metadata": {},
|
||
|
"outputs": [],
|
||
|
"source": [
|
||
|
"# pd.plotting.scatter_matrix(df[[\"vib\", \"distance\", \"projection\", \"v-radial\", \"v-tang\"]], alpha=0.5)\n",
|
||
|
"plt.show()"
|
||
|
]
|
||
|
},
|
||
|
{
|
||
|
"cell_type": "code",
|
||
|
"execution_count": 80,
|
||
|
"metadata": {},
|
||
|
"outputs": [],
|
||
|
"source": [
|
||
|
"# bins = np.linspace(0, df['v-radial'].max(), 10)\n",
|
||
|
"# df[\"binned-v-radial\"] = pd.cut(df['v-radial'], bins)\n",
|
||
|
"# df.groupby(\"binned-v-radial\").agg({\"vib\": {\"min\", \"median\", \"mean\", \"max\", \"count\"}})"
|
||
|
]
|
||
|
},
|
||
|
{
|
||
|
"cell_type": "code",
|
||
|
"execution_count": 81,
|
||
|
"metadata": {},
|
||
|
"outputs": [],
|
||
|
"source": [
|
||
|
"# corr = df[df.columns.sort_values()].corr()[[\"vib\", \"vib-x\", \"vib-y\"]]\n",
|
||
|
"# cm = sns.light_palette(\"orange\", as_cmap=True) \n",
|
||
|
"# cm = sns.diverging_palette(220, 20, sep=20, as_cmap=True) \n",
|
||
|
"# corr.style.background_gradient(cmap=cm).set_precision(2)"
|
||
|
]
|
||
|
},
|
||
|
{
|
||
|
"cell_type": "code",
|
||
|
"execution_count": null,
|
||
|
"metadata": {},
|
||
|
"outputs": [],
|
||
|
"source": []
|
||
|
},
|
||
|
{
|
||
|
"cell_type": "code",
|
||
|
"execution_count": 82,
|
||
|
"metadata": {},
|
||
|
"outputs": [
|
||
|
{
|
||
|
"data": {
|
||
|
"text/markdown": [
|
||
|
"\n",
|
||
|
"## Inline Description:\n",
|
||
|
"\n",
|
||
|
"It is very nice to describe results using Markdown with dynamic values like: **3.141592653589793**.\n",
|
||
|
"\n"
|
||
|
],
|
||
|
"text/plain": [
|
||
|
"<IPython.core.display.Markdown object>"
|
||
|
]
|
||
|
},
|
||
|
"execution_count": 82,
|
||
|
"metadata": {},
|
||
|
"output_type": "execute_result"
|
||
|
}
|
||
|
],
|
||
|
"source": [
|
||
|
"from IPython.display import Markdown \n",
|
||
|
"Markdown(\"\"\"\n",
|
||
|
"## Inline Description:\n",
|
||
|
"\n",
|
||
|
"It is very nice to describe results using Markdown with dynamic values like: **{pi}**.\n",
|
||
|
"\n",
|
||
|
"\"\"\".format(pi=np.pi))"
|
||
|
]
|
||
|
},
|
||
|
{
|
||
|
"cell_type": "markdown",
|
||
|
"metadata": {},
|
||
|
"source": [
|
||
|
"# Machine Learning\n",
|
||
|
"\n",
|
||
|
"**Be careful when handling with Artificial Intelligence:**\n",
|
||
|
"\n",
|
||
|
"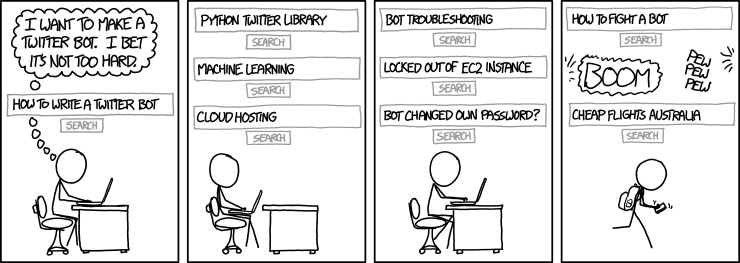\n"
|
||
|
]
|
||
|
},
|
||
|
{
|
||
|
"cell_type": "code",
|
||
|
"execution_count": null,
|
||
|
"metadata": {},
|
||
|
"outputs": [],
|
||
|
"source": []
|
||
|
}
|
||
|
],
|
||
|
"metadata": {
|
||
|
"kernelspec": {
|
||
|
"display_name": "Python 3",
|
||
|
"language": "python",
|
||
|
"name": "python3"
|
||
|
},
|
||
|
"language_info": {
|
||
|
"codemirror_mode": {
|
||
|
"name": "ipython",
|
||
|
"version": 3
|
||
|
},
|
||
|
"file_extension": ".py",
|
||
|
"mimetype": "text/x-python",
|
||
|
"name": "python",
|
||
|
"nbconvert_exporter": "python",
|
||
|
"pygments_lexer": "ipython3",
|
||
|
"version": "3.7.3"
|
||
|
}
|
||
|
},
|
||
|
"nbformat": 4,
|
||
|
"nbformat_minor": 4
|
||
|
}
|